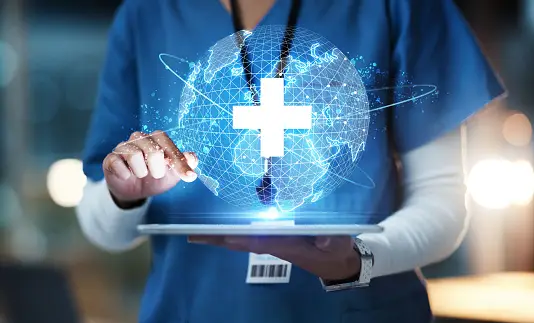
Artificial Intelligence’s Effect on Medical Diagnostics In 2023? As of my last knowledge update in January 2023, I can provide you with a general overview of how artificial intelligence (AI) has been influencing medical diagnostics up to that point. Keep in mind that specific advancements and changes may have occurred since then.
Table of Contents
ToggleMedical Imaging
Improved Accuracy: AI algorithms have been increasingly integrated into medical imaging technologies, such as MRI, CT scans, and X-rays, to enhance diagnostic accuracy. AI systems can analyze medical images with a high level of precision, assisting radiologists in detecting abnormalities and diseases.
Faster Analysis: AI-powered image analysis can significantly reduce the time required for diagnostics. Rapid identification of anomalies allows for quicker decision-making in patient care.
Pathology and Histology
Automated Histopathology: AI is aiding pathologists in analyzing tissue samples by automating certain tasks, like identifying and classifying cells. This speeds up the diagnostic process and helps ensure consistency in results.
Cancer Detection: AI algorithms are being utilized for early detection of cancers by analyzing pathology slides. This has the potential to enhance the chances of successful treatment through early intervention.
Predictive Analytics
Risk Prediction: AI is used to analyze patient data to predict the likelihood of developing certain medical conditions. This can enable proactive measures and personalized treatment plans for individuals at higher risk.
Genomic Medicine
Precision Medicine: AI plays a role in analyzing vast genomic datasets to identify genetic markers associated with diseases. This information is used to tailor treatment plans to an individual’s genetic makeup, enhancing the effectiveness of therapies.
Natural Language Processing (NLP)
Data Extraction from Clinical Notes: NLP is employed to extract valuable information from unstructured clinical notes and patient records. This assists healthcare providers in gaining insights from a wealth of patient data.
Virtual Health Assistants
Patient Interaction: AI-driven virtual assistants are being used to interact with patients, gather medical histories, and provide initial diagnostic information. This improves patient engagement and streamlines the collection of relevant health information.
-
Top AI Applications in Healthcare for 202525 January 2025
-
Key Tech Innovations Reshaping Healthcare in 202510 January 2025
Remote Monitoring
Continuous Monitoring: AI facilitates remote monitoring of patients with chronic conditions. Wearable devices equipped with AI can track vital signs and provide real-time data to healthcare providers, enabling proactive intervention.
Challenges and Considerations
Data Privacy and Security: The use of AI in medical diagnostics raises concerns about the privacy and security of patient data. Robust measures are required to safeguard sensitive health information.
Regulatory Frameworks: Developing and updating regulatory frameworks to ensure the safe and ethical use of AI in healthcare is an ongoing challenge.
In 2023, the integration of AI into medical diagnostics is likely to continue advancing, with ongoing research and development aimed at addressing existing challenges and expanding the range of applications. Please verify with more recent sources for the latest developments.
Read More: Exploring the Benefits of Health Insurance In 2023
Interoperability and Integration
Integration of AI Systems: Efforts are underway to seamlessly integrate AI systems into existing healthcare infrastructure. This includes compatibility with electronic health record (EHR) systems, ensuring that AI-generated insights can be easily accessed and utilized by healthcare professionals.
Explainable AI (XAI)
Transparency in Decision-Making: As AI systems become more complex, the need for transparency in their decision-making processes is recognized. Explainable AI aims to provide insights into how AI algorithms reach specific conclusions, enhancing trust among healthcare providers and patients.
Collaboration and Knowledge Sharing
International Collaboration: The global healthcare community is increasingly collaborating on AI research and implementation. Sharing knowledge and best practices helps accelerate the development of effective AI solutions and ensures that advancements benefit diverse populations.
Education and Training
Healthcare Professional Training: With the integration of AI, there is a growing need for training healthcare professionals in understanding and effectively utilizing AI tools. Educational programs are being developed to ensure that clinicians can leverage AI for improved diagnostics and patient care.
Ethical Considerations
Bias Mitigation: Addressing biases in AI algorithms is a critical concern. Efforts are being made to identify and mitigate biases that could disproportionately affect certain patient groups, ensuring that AI applications are fair and equitable.
Real-Time Decision Support
Clinical Decision Support Systems: AI is increasingly used in real-time decision support for healthcare professionals. This involves providing clinicians with relevant information and recommendations during the diagnostic and treatment process, contributing to more informed decision-making.
Continuous Learning and Adaptation
Adaptive Algorithms: AI systems are being designed to continuously learn and adapt based on new data and experiences. This dynamic nature allows the algorithms to improve over time, staying current with the latest medical knowledge and trends.
Cost and Accessibility
Affordability and Accessibility: Efforts are being made to develop cost-effective AI solutions to ensure broader accessibility, especially in resource-limited settings. This includes exploring the use of AI in low-resource environments to enhance diagnostic capabilities.
Regulatory Advancements
Adaptive Regulations
Regulatory bodies are working to adapt to the rapidly evolving field of AI in healthcare. Establishing clear guidelines and standards is crucial to ensure the safe and effective deployment of AI technologies in medical diagnostics.
Conclusion
the integration of artificial intelligence into medical diagnostics in 2023 is characterized by rapid advancements, increased collaboration, and a growing focus on ethical considerations and transparency. While challenges persist, ongoing research and a commitment to addressing these challenges indicate a promising future for AI in revolutionizing healthcare and improving patient outcomes. It’s essential to stay updated with the latest developments to understand the evolving landscape of AI in medical diagnostics.
FAQs
What is artificial intelligence in medical diagnostics?
Artificial intelligence (AI) in medical diagnostics refers to the use of advanced algorithms and computational techniques to analyze medical data, including imaging, pathology, and patient records, to assist healthcare professionals in diagnosis and treatment decisions.
How does AI improve medical diagnostics?
AI improves medical diagnostics by enhancing the accuracy and efficiency of tasks such as image analysis, pathology interpretation, and risk prediction. It can assist healthcare professionals in identifying patterns and anomalies in data, leading to quicker and more accurate diagnoses.
What types of medical imaging benefit from AI?
AI is applied to various medical imaging modalities, including MRI, CT scans, X-rays, and ultrasound. It aids in image interpretation, detection of abnormalities, and the overall improvement of diagnostic capabilities.
Can AI replace human doctors in medical diagnostics?
No, AI is designed to complement and assist healthcare professionals, not replace them. AI systems can analyze data and provide valuable insights, but human expertise is crucial for interpreting complex information, considering patient context, and making treatment decisions.
Are there ethical concerns with the use of AI in medical diagnostics?
Yes, ethical concerns include issues related to patient privacy, data security, potential biases in algorithms, and the responsible use of AI-generated insights. Efforts are made to address these concerns through regulations, guidelines, and transparent practices.
How is AI used in pathology and histology?
AI in pathology and histology involves automating tasks such as cell identification and classification in tissue samples. This speeds up the analysis process and contributes to more accurate diagnoses, especially in the detection of cancer and other diseases.
What is Explainable AI (XAI) in medical diagnostics?
Explainable AI (XAI) refers to the transparency and interpretability of AI algorithms. In medical diagnostics, XAI aims to provide insights into how AI systems reach specific conclusions, making the decision-making process more understandable for healthcare professionals and patients.
How does AI contribute to precision medicine?
AI contributes to precision medicine by analyzing large genomic datasets to identify genetic markers associated with diseases. This information is used to tailor treatment plans based on an individual’s genetic makeup, increasing the effectiveness of therapies.
What challenges does AI face in medical diagnostics?
Challenges include data privacy and security, addressing biases in algorithms, regulatory compliance, integration with existing healthcare systems, and ensuring the ethical use of AI in patient care.